Biomarkers of diabetic retinopathy
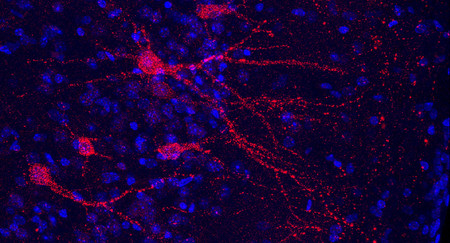
image of cells
Combining non-imaging and imaging-based models for better detection of diabetic retinopathy.
An ensemble learning approach that enables better detection, prediction and assessment of diabetic retinopathy.
The challenge
Diabetic retinopathy remains the leading cause of acquired vision loss and blindness in the middle-aged population.
Until now, artificial intelligence (AI) based diabetic retinopathy (DR) grading systems have explicitly used information available on photographs of the fundus (the part of the eye opposite the pupil) to determine disease category.
Few attempts have been made to automatically determine DR using other non-imaging biomarkers that have a strong correlation with DR.
The goal is to develop novel DR assessment procedures to reduce the burden of DR.
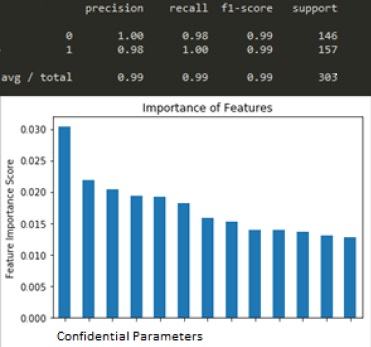
Novel machine learning methods have been developed to use non-imaging biomarkers for independent risk assessment of diabetic retinopathy.
Our response
We have developed an AI system that combines imaging and non-imaging biomarkers for better detection and assessment of diabetic retinopathy. The system employs deep learning methodology to use colour fundus photographs to grade DR.
An ensemble learning model that combines both non-imaging and imaging-based decisions has also been developed.
Benefits
DR has been independently associated with decreased quality of life and poses a significant financial burden on society through the loss of productivity and earning capacity.
Our proposed AI-based system contributes to 5 per cent more accurate detection of DR compared to using image-only modality.
It’s hoped that improved assessment of the disease will contribute to reducing blindness, improving sufferers’ quality of life, and reducing the cost burden.
The Australian e-Health Research Centre (AEHRC) is CSIRO's digital health research program and a joint venture between CSIRO and the Queensland Government. The AEHRC works with state and federal health agencies, clinical research groups and health businesses around Australia.